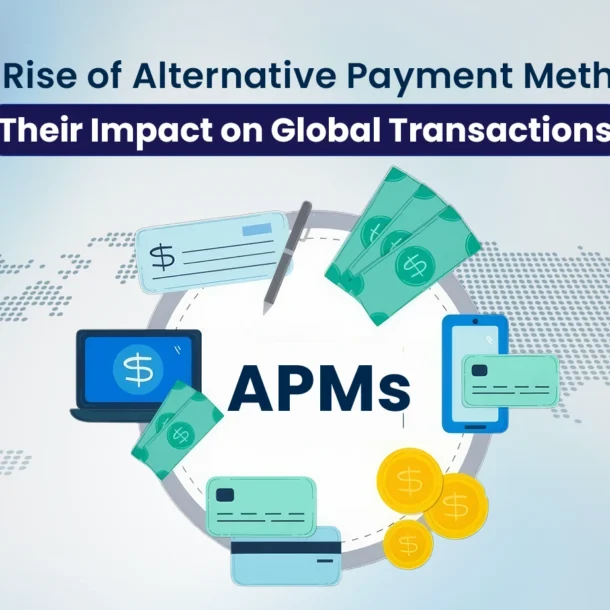
The fintech industry is experiencing rapid growth as digital payments, mobile banking, and decentralized financial services become increasingly popular. With this growth, however, comes a significant rise in fraudulent activities, which pose a serious threat to both businesses and customers. Traditional fraud detection methods are becoming obsolete as fraudsters develop more advanced techniques to exploit weaknesses in financial systems. This is where artificial intelligence (AI) and machine learning (ML) are proving to be game-changers, reshaping how fintech companies detect and prevent fraud.
Fraud prevention in fintech is no longer just about flagging suspicious transactions; it’s about creating systems that can learn and adapt in real time. AI and ML empower fintech firms to mitigate risks more effectively, enhance customer security, and streamline operations.
The adoption of digital banking has revolutionized the financial sector, allowing for faster transactions, more convenience, and broader accessibility. However, with the surge in online banking and digital wallets, fintech companies face new security challenges. Fraudsters are targeting digital platforms through identity theft, phishing scams, and account takeovers, which can cause significant financial and reputational damage.
For instance, payment fraud has become a major concern as criminals exploit payment gateways, creating fake accounts and orchestrating fraud schemes through bot attacks. The global shift toward contactless and cashless payments has also contributed to an increase in fraudulent transactions.
In the past, traditional fraud detection methods relied heavily on rule-based systems. These systems detect fraud based on predefined thresholds and static patterns, such as transactions above a certain amount or transfers made from suspicious locations. However, modern fraudsters have become adept at bypassing these systems by leveraging more sophisticated methods, including synthetic identities and social engineering attacks.
As fintech platforms scale globally, the complexity of fraud schemes has grown exponentially. Traditional fraud prevention tools struggle to keep pace, which is why AI and machine learning are now vital to detecting and preventing fraud in real-time.
The ability to analyze large volumes of data is one of the key advantages of AI-powered fraud prevention systems. Fintech companies collect vast amounts of customer data, including transaction histories, account behaviors, geographic information, and more. AI and machine learning algorithms can sift through this data to identify subtle anomalies that may indicate fraud.
For example, if a customer’s transaction behavior suddenly deviates from the norm—such as making a large transaction from an unfamiliar location—AI systems can flag this as suspicious. Machine learning models use historical data to “learn” what constitutes normal versus abnormal behavior, improving the accuracy of fraud detection.
Traditional systems often lag in detecting fraudulent transactions, leading to delayed responses and greater financial losses. In contrast, AI and machine learning enable real-time threat detection, allowing fintech companies to respond immediately to potential fraud.
Real-time detection is particularly useful for spotting phishing attacks, account takeovers, and card-not-present (CNP) fraud. By continuously monitoring transactions and user behavior, AI can identify fraudulent activity within milliseconds, minimizing potential losses.
Predictive analytics is another critical feature of AI-driven fraud prevention systems. By analyzing historical data and identifying patterns that precede fraud attempts, machine learning models can predict when and where fraud is likely to occur. This proactive approach allows fintech companies to take preemptive actions to mitigate risks.
For example, a predictive model might recognize that a certain user segment is more vulnerable to phishing attacks based on past behavior. Fintech companies can use this information to tighten security protocols, such as implementing two-factor authentication (2FA) or issuing warnings to high-risk customers.
False positives occur when legitimate transactions are incorrectly flagged as fraudulent, leading to customer frustration and potential loss of business. AI and machine learning help reduce false positives by refining fraud detection models. Unlike rule-based systems, AI can personalize fraud detection for each customer, reducing the likelihood of errors.
For instance, AI can differentiate between a legitimate transaction made by a customer traveling abroad and a fraudulent one by analyzing multiple data points, such as the user’s travel history, spending patterns, and device information.
In addition to preventing fraud, AI and machine learning enhance the overall customer experience. Traditional fraud prevention systems can be disruptive, requiring customers to verify their identity or re-enter their information for every flagged transaction. AI-driven systems are less intrusive, providing seamless security in the background without disrupting the user experience.
Moreover, with AI, companies can offer personalized security measures, such as biometric authentication or behavioral analysis, to ensure customers feel protected without being inconvenienced.
As fintech companies grow, so does the volume of transactions they must process. AI-powered fraud prevention tools can easily scale to accommodate this growth, ensuring that fraud detection systems remain effective regardless of transaction volume. This scalability is essential for companies operating in multiple regions, as AI models can be trained to detect region-specific fraud patterns.
One of the most significant use cases for AI in fintech is detecting identity fraud. Fraudsters often use synthetic identities or stolen credentials to gain access to financial services. AI models can analyze customer data, such as behavior patterns, biometrics, and document authenticity, to detect fraudulent attempts during the onboarding process.
For instance, AI systems can cross-reference a user’s data with public records or use facial recognition technology to verify the authenticity of identity documents, reducing the likelihood of fraud during account creation.
AI and machine learning can monitor payment systems for anomalies that indicate potential fraud. Machine learning algorithms are capable of recognizing irregular transaction patterns that could signal a fraudulent attempt, such as multiple small transactions followed by a large one, or unusual geographical spending patterns.
Account takeovers are one of the fastest-growing types of fraud in the fintech industry. AI-based systems can help prevent ATOs by analyzing login behaviors, such as device type, IP address, and login location. If suspicious behavior is detected, AI systems can trigger additional security measures, such as requiring biometric authentication or alerting the user.
Natural language processing (NLP) is playing an increasingly important role in fraud prevention. NLP algorithms can analyze text-based communications—such as emails, chat messages, and social media posts—to detect phishing attempts, scam emails, and other social engineering tactics used by fraudsters.
Explainable AI is a trend that addresses the “black box” nature of many AI systems. In fintech, regulatory compliance requires that companies understand and explain the decisions made by their AI models. Explainable AI helps fintech firms meet these requirements by providing transparent, interpretable insights into how fraud detection algorithms arrive at their conclusions.
The integration of AI with blockchain technology is an emerging area of interest in fintech. AI can enhance blockchain’s ability to prevent fraud by continuously monitoring the blockchain ledger for unusual activities, ensuring the security and transparency of digital transactions.
By adopting AI and machine learning-based fraud prevention tools, fintech firms can not only protect their customers but also enhance the overall user experience, reduce operational costs, and maintain trust in an increasingly digital financial world.
AI in fraud prevention refers to the use of artificial intelligence systems to detect, analyze, and prevent fraudulent activities in real-time. By leveraging machine learning algorithms, AI systems can process large volumes of transaction data to identify suspicious patterns and anomalies that may indicate fraud.
Machine learning enhances fraud detection by continuously learning from past data and improving the accuracy of identifying fraud patterns. Unlike traditional rule-based systems, machine learning models can adapt to new and evolving fraud techniques, detecting abnormal behavior that traditional systems might miss.
AI reduces false positives by analyzing multiple data points, such as user behavior, transaction history, and device information. Machine learning models can personalize fraud detection for each user, minimizing the chances of flagging legitimate transactions as fraudulent.
Businesses benefit from AI-driven fraud prevention by reducing financial losses due to fraud, improving customer trust and loyalty, enhancing operational efficiency, and maintaining compliance with evolving security standards.
AI-powered systems can easily scale to handle increased transaction volumes as fintech companies grow. Machine learning models can be trained to detect fraud across different regions, adapting to local fraud patterns and evolving with the company’s needs.